The automotive industry is undergoing a profound transformation, driven by the rapid advancement of technologies such as Artificial Intelligence (AI) and Machine Learning (ML). In particular, the integration of these technologies in automotive molding—a critical manufacturing process for producing components like bumpers, dashboards, and interior trims—is revolutionizing production efficiency, quality control, and product innovation.
1. Understanding Automotive Molding
Automotive molding involves shaping materials—typically plastics or composites—into precise parts using molds under high pressure and temperature. These parts are essential for vehicle design, safety, and performance. Traditional molding methods, while effective, often suffer from issues such as:
Material waste
Quality inconsistencies
Prolonged cycle times
High defect rates
2. Role of AI and Machine Learning
AI and ML technologies are being increasingly deployed to optimize various stages of the molding process:
a. Predictive Maintenance
Machine learning models analyze sensor data from molding equipment to predict potential failures before they occur. This helps minimize downtime and maintenance costs by enabling timely interventions.
b. Process Optimization
AI systems monitor and control parameters like temperature, pressure, and cooling time in real-time to optimize the molding process. Algorithms continuously learn from production data to improve cycle times and reduce energy consumption.
c. Defect Detection and Quality Assurance
Computer vision powered by AI can detect surface defects such as warping, bubbles, or sink marks far more accurately than manual inspection. ML models can also correlate production parameters with defect types, enabling root-cause analysis and proactive corrections.
d. Material Flow Simulation
AI-driven simulation tools help predict how materials will flow and cool inside the mold, allowing engineers to adjust designs and settings for better performance, reduced material use, and fewer defects.
3. Benefits of AI and ML Integration
Enhanced Product Quality: Continuous monitoring and optimization reduce inconsistencies and improve part precision.
Lower Costs: Automation and predictive analytics cut down on scrap, rework, and unplanned downtime.
Shorter Development Cycles: Smart simulation tools accelerate mold design and testing phases.
Sustainability: Reduced material waste and energy consumption contribute to greener manufacturing practices.
4. Real-World Applications
Leading automotive manufacturers and suppliers are already leveraging AI in molding operations. Examples include:
Bosch: Using ML algorithms for mold tool wear prediction and maintenance scheduling.
Toyota: Implementing AI for inline inspection and real-time quality feedback loops.
Magna International: Employing data-driven molding process adjustments to reduce cycle time and defects.
5. Challenges and Future Outlook
Despite its benefits, the adoption of AI in automotive molding faces hurdles such as:
Data integration from legacy systems
High initial investment in AI infrastructure
Need for skilled personnel in AI and data science
However, as digital transformation accelerates, these challenges are expected to diminish. The future holds promising advancements, including the use of generative AI for mold design, edge AI for real-time control at the machine level, and autonomous molding systems capable of self-adjustment and learning.
Conclusion
AI and machine learning are no longer optional in the competitive landscape of automotive manufacturing—they are critical enablers of efficiency, quality, and innovation. By embedding intelligence into molding processes, automotive companies can not only optimize operations but also deliver safer, more reliable, and cost-effective vehicles to the market.
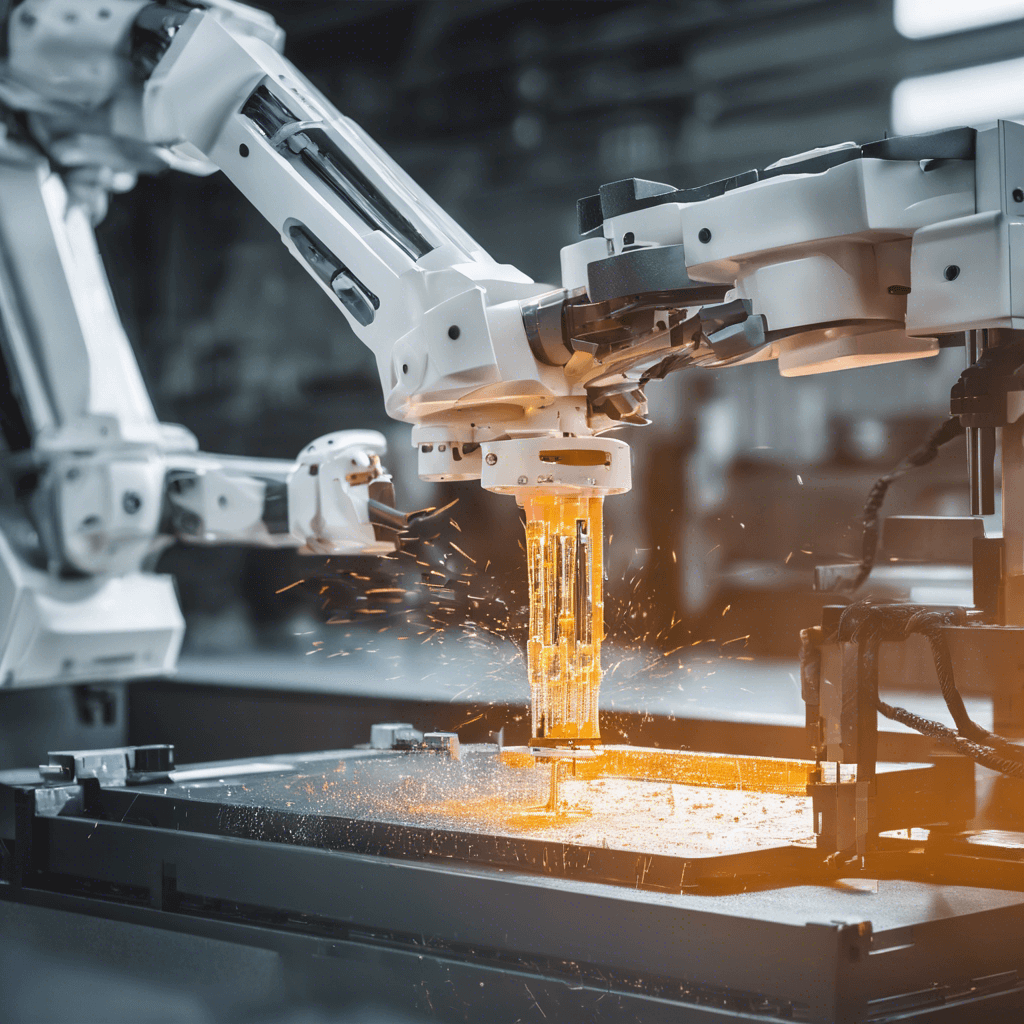