Injection molding is a widely used manufacturing process for producing plastic parts by injecting molten material into a mold. As manufacturing technologies advance, integrating Artificial Intelligence (AI) and Machine Learning (ML) into injection molding is revolutionizing how parts are designed, produced, and optimized.
1. Role of AI and ML in Injection Molding
AI and ML enhance the efficiency, precision, and quality of injection molding processes. Their primary roles include:
Process Optimization: AI algorithms can automatically adjust parameters like pressure, temperature, and cooling time to reduce defects and cycle times.
Predictive Maintenance: ML models analyze equipment usage and sensor data to predict failures before they happen, reducing downtime.
Quality Control: Image recognition and anomaly detection algorithms can identify defects in real-time with greater accuracy than manual inspection.
Material Optimization: AI helps determine the best materials or additives for specific product requirements and manufacturing conditions.
Design for Manufacturability (DFM): AI-driven simulation tools assist in optimizing part and mold design before production begins.
2. Key Applications
a. Real-Time Monitoring and Control
Machine learning models analyze real-time data from sensors embedded in the molding machines to:
Detect process deviations.
Adjust parameters on the fly.
Maintain consistent product quality.
b. Defect Detection
Computer vision systems powered by AI can inspect parts immediately after molding to identify:
Short shots
Sink marks
Warpage
Flash and other surface defects
c. Predictive Analytics
By learning from historical production data, ML models predict:
Equipment failure times
Maintenance needs
Expected yield and production efficiency
d. Process Simulation and Optimization
AI-driven simulations help engineers:
Predict material flow and cooling patterns.
Optimize gate placement, runner systems, and cycle times.
Reduce the number of physical prototypes.
3. Benefits
Improved Product Quality: Reduced defects and rework through intelligent monitoring.
Cost Reduction: Less material waste and lower labor costs through automation and efficient resource use.
Faster Time to Market: Optimized designs and processes speed up development cycles.
Greater Process Stability: Consistent and repeatable quality with reduced variability.
4. Challenges
Data Collection & Management: High-quality, clean data is essential for effective AI/ML modeling.
Integration with Legacy Systems: Older molding machines may not support modern sensors or data outputs.
Skill Gap: Requires staff with expertise in AI, data science, and process engineering.
Initial Investment: Costs of AI tools, sensors, and training can be high, although ROI is typically strong over time.
5. Future Outlook
Smart Factories: AI-driven injection molding will become a core part of Industry 4.0, with fully connected, autonomous production lines.
Edge Computing: Real-time decisions at the machine level without cloud latency.
Self-Healing Systems: Molding machines that self-diagnose and self-correct.
Sustainable Manufacturing: AI will help optimize energy and material usage for greener operations.
Conclusion
The integration of AI and Machine Learning in injection molding is transforming traditional manufacturing into a smart, adaptive, and efficient system. With the right strategy, businesses can unlock significant benefits in quality, speed, and cost-effectiveness.
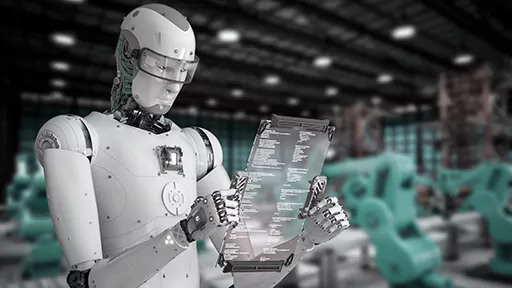