PVC (Polyvinyl Chloride) injection molding is a widely used manufacturing process for producing durable plastic parts with complex geometries. However, it involves multiple variables—temperature, pressure, cooling time, injection speed, etc.—that must be precisely controlled to ensure consistent product quality. Traditional methods of process control often rely on trial and error or static parameters, which can lead to inefficiencies, higher defect rates, and material waste.
Artificial Intelligence (AI) offers a transformative solution by enabling data-driven, real-time optimization. By integrating AI technologies into PVC injection molding, manufacturers can significantly enhance efficiency, reduce cycle times, and improve product quality.
Key Components of AI-Driven Optimization
1. Data Collection and Sensor Integration
Modern molding machines are equipped with sensors that track parameters like:
Mold temperature
Injection pressure
Material viscosity
Machine vibrations
Cycle time
This real-time data serves as the foundation for AI models to detect patterns and anomalies.
2. Machine Learning Models
AI algorithms, particularly machine learning (ML), are used to:
Predict optimal process settings
Identify and prevent defects (e.g., warping, burn marks, bubbles)
Estimate maintenance needs based on machine usage and sensor readings
Common ML models applied include:
Regression models for process prediction
Classification algorithms for defect detection
Reinforcement learning for adaptive control
3. Process Optimization
AI can dynamically adjust process parameters to maintain optimal conditions. Examples include:
Adjusting cooling time based on mold temperature trends
Tuning injection speed to avoid air entrapment
Modifying holding pressure to prevent sink marks
These adjustments improve part consistency and reduce scrap rates.
4. Predictive Maintenance
AI systems analyze historical and real-time data to forecast machine component wear and potential failures. This helps in:
Minimizing downtime
Reducing unplanned maintenance
Extending equipment lifespan
Benefits of AI in PVC Injection Molding
Improved Product Quality: AI helps in identifying defects early, maintaining tight tolerances, and reducing human error.
Increased Efficiency: Real-time optimization lowers cycle times and energy usage.
Reduced Waste: Fewer defective parts mean less material waste and rework.
Adaptive Learning: AI models improve over time with more data, increasing process robustness.
Enhanced Process Control: AI offers better insights and control than traditional statistical process control methods.
Challenges and Considerations
Data Quality: Accurate, high-resolution data is critical for effective AI performance.
Integration Complexity: Retrofitting AI systems into existing equipment can be technically demanding.
Skilled Workforce: Operators and engineers need training to interpret AI recommendations and maintain systems.
Cost: Initial investment in AI infrastructure can be significant, though it often leads to long-term ROI.
Future Outlook
AI in PVC injection molding is evolving toward fully autonomous systems, where human oversight is minimal. Integration with Industrial IoT (IIoT), digital twins, and cloud-based analytics will further enhance predictive capabilities, global scalability, and remote diagnostics. As AI models become more sophisticated, they will enable manufacturers to move from reactive to proactive and even prescriptive process management.
Conclusion
AI-driven optimization in PVC injection molding is revolutionizing how manufacturers approach quality, efficiency, and sustainability. By embracing intelligent systems, businesses can unlock higher productivity, lower costs, and a competitive edge in a rapidly evolving industry.
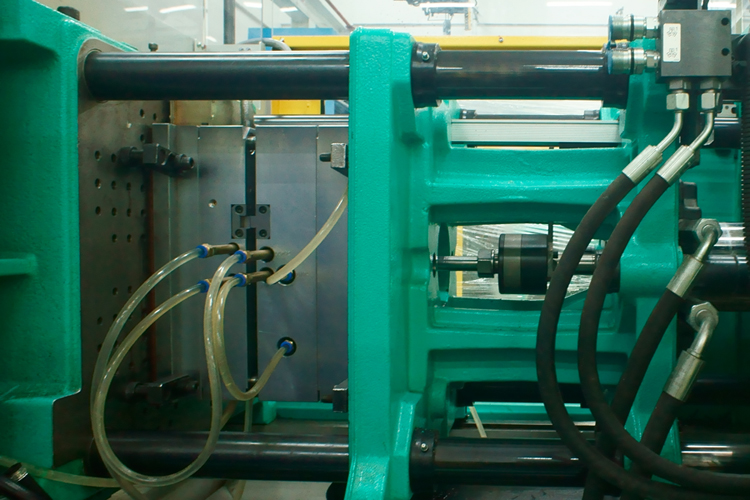