Injection molding is a critical manufacturing process used to produce high-precision plastic parts. However, it involves numerous variables such as temperature, pressure, material flow, and cooling time, which need to be precisely controlled for quality and efficiency. A Digital Twin offers a powerful solution for optimizing this complex process.
What is a Digital Twin?
A Digital Twin is a virtual replica of a physical system that integrates real-time data and simulations to replicate, analyze, and optimize the system’s behavior. In the context of injection molding, a digital twin can model every stage of the molding process, from mold design to machine operation and part quality.
Benefits of Using Digital Twin in Injection Molding
Process Optimization
Enables simulation of various process parameters (e.g., injection speed, melt temperature) to identify optimal settings.
Reduces trial-and-error iterations on the physical machine.
Real-Time Monitoring and Control
Sensors embedded in the molding machine feed live data to the digital twin.
Predicts deviations and allows dynamic adjustments to maintain consistent part quality.
Predictive Maintenance
Identifies wear and tear in molds or equipment based on operational data.
Helps schedule maintenance before failures occur, reducing downtime.
Defect Reduction
Simulates and predicts defects such as warping, sink marks, or short shots.
Adjusts parameters proactively to minimize scrap rate.
Faster Time-to-Market
Streamlines mold design validation through virtual prototyping.
Reduces the need for physical samples and iterative testing.
Sustainability and Cost Savings
Optimizes energy usage and reduces material waste.
Leads to lower production costs and improved environmental footprint.
Core Components of a Digital Twin for Injection Molding
3D CAD Model of Mold and Product: Forms the basis for simulation and analysis.
Process Simulation Tools: Software like Autodesk Moldflow or SIGMASOFT for simulating filling, cooling, and packing stages.
Sensor Data Integration: Real-time data from temperature, pressure, and displacement sensors.
Analytics and AI Algorithms: For anomaly detection, trend analysis, and process optimization.
IoT & Cloud Platforms: Enable remote monitoring, data storage, and advanced computation.
Implementation Challenges
High Initial Investment: Cost of sensors, software, and integration may be a barrier for small to medium enterprises.
Data Management Complexity: Handling large volumes of real-time data requires robust infrastructure.
Skill Gaps: Requires expertise in both manufacturing and digital technologies.
Case Study Example (Optional Section)
A leading automotive parts manufacturer implemented a digital twin for a multi-cavity injection molding machine. Using real-time pressure and temperature data combined with AI-driven simulation, the company reduced scrap by 25% and improved cycle time by 12%.
Future Outlook
As technologies like AI, machine learning, and edge computing continue to evolve, digital twins in injection molding will become even more intelligent and autonomous. Integration with enterprise systems like ERP and PLM will offer end-to-end visibility and control across the production lifecycle.
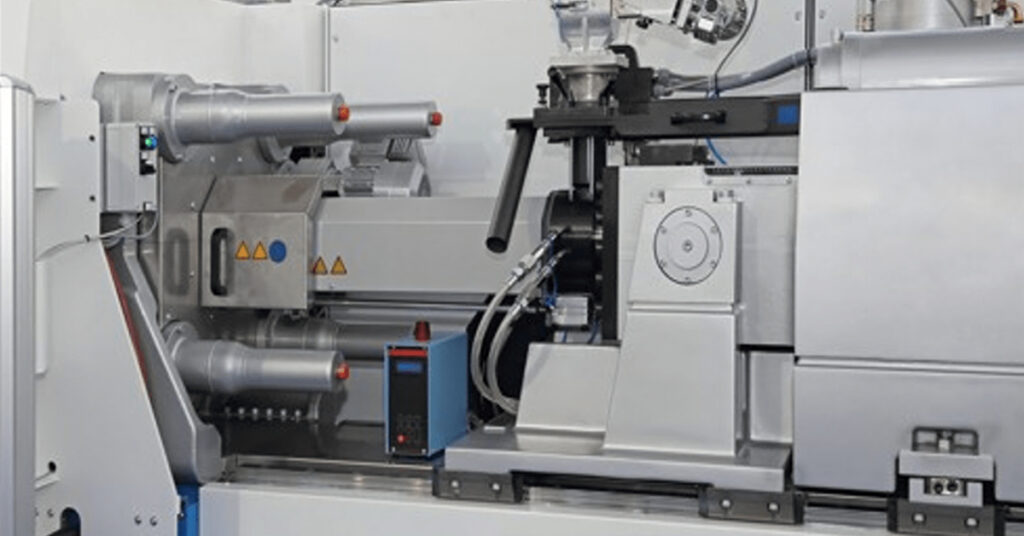